Research
Preoperative Mapping using Resting-State fMRI
Neurosurgery remains the most common treatment option for the 100,000 people per year diagnosed with a primary brain tumor in the United States. Long-term outcomes for these patients are governed by two competing factors: aggressiveness of the tumor resection and preservation of sensorimotor and language functionality in the brain known as the eloquent cortex. Task fMRI has emerged as a popular tool for preoperative mapping; it uses carefully-designed experimental paradigms to activate the relevant areas of the eloquent cortex. While noninvasive, t-fMRI is unreliable in many brain tumor patients, who struggle to perform these cognitively demanding tasks.
This project develops the clinical potential of resting-state fMRI (rs-fMRI) for eloquent cortex mapping. Rs-fMRI captures steady-state patterns of co-activation in the brain associated with different functional systems. Importantly, it does not require behavioral paradigms and can be performed while the patient is sleeping or under mild anesthesia, thus overcoming many of the practical challenges of task fMRI. We have developed novel deep learning architectures for rs-fMRI that leverage topologically constrained row and column filters, designed to operate on the co-activation (i.e., connectivity) inputs. Our models achieve high sensitivity and specificity, while being robust to data subsampling. We are now moving towards dynamic analyses using spatial and temporal attention layers and the integration of structural connectivity information via diffusion MRI.
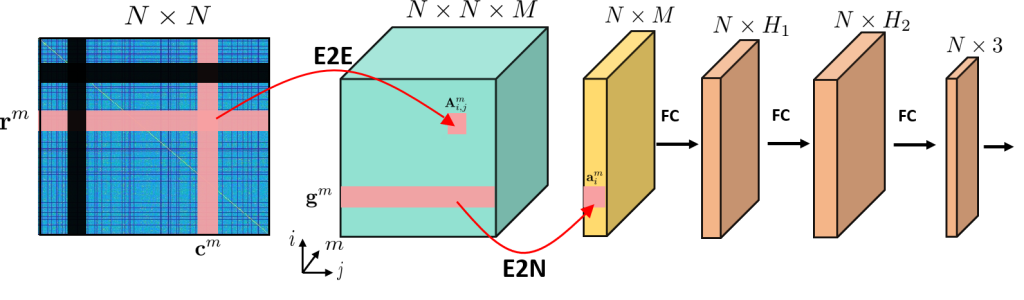
SELECTED PUBLICATIONS
Automated Eloquent Cortex Localization in Brain Tumor Patients Using Multi-task Graph Neural Networks.
N. Nandakumar, K. Manzoor, S. Agarwal, J. Pillai, S. Gujar, H. Sair, A. Venkataraman.
Medical Image Analysis (MedIA), 74:102203, 2021.
A Multi-Scale Spatial and Temporal Attention Network on Dynamic Connectivity to Localize The Eloquent Cortex in Brain Tumor Patients.
N. Nandakumar, K. Manzoor, S. Agarwal, J. Pillai, S. Gujar, H. Sair, A. Venkataraman.
In Proc. IPMI: Information Processing in Medical Imaging, LNCS 12729:241-252, 2021. [Acceptance Rate ≈ 30%]
A Multi-Task Deep Learning Framework to Localize the Eloquent Cortex in Brain Tumor Patients using Both Static and Dynamic Functional Connectivity.
N. Nandakumar, N.S. D’Souza, K. Manzoor, J. Pillai, S. Gujar, S. Agarwal, H. Sair, A. Venkataraman.
In Proc. MLCN: MICCAI Workshop on Machine Learning for Clinical Neuroimaging, LNCS 12449:34-44, 2020. Selected for an Oral Presentation – Best Paper Award
A Novel Graph Neural Network to Localize Eloquent Cortex in Brain Tumor Patients from Resting-State fMRI Connectivity.
N. Nandakumar, K. Manzoor, J. Pillai, S. Gujar, H. Sair, A. Venkataraman.
In Proc. CNI: MICCAI Workshop on Connectomics in Neuroimaging, LNCS 11848:10-20, 2019. Selected for an Oral Presentation (<25% of Papers) – Best Paper Award
FUNDING